| a summary on first thoughts and findings on my possible master thesis topic
While I was continuing my research about different in-vehicle interface solutions and future trends, it became clear for me, that driver assistance systems and “autopilot” (autonomous driving) functions play a major role in the cockpits’ features. By assistance systems I mean on the one hand features like lane-keeping, speed control, parking and front distance controls, and on the other hand speaking assistants like Siri or Alexa built in to control navigation and other features. When thinking about the needs of interfaces and the human-machine-interaction with these assistants, for me the most interesting topic is how to get humans to trust the machine that they give the control over?
If Alexa cannot tell the exact weather outside or doesn’t find the song you want to hear, you forgive her and try another time. But if your car does an emergency braking without any reason or does not stop at a red light in autonomous mode, possibly threatening your life, you won’t forgive it and will probably never hand over the control again.
These are my thoughts why I would like to research this topic further:
How can we create trust in the vehicles’ assistance systems via interfaces and newest technologies, like augmented reality?
With carrying out case studies, user surveys and user testing of different concepts about existing solutions and new proposals, whether they help to build trust or not, I could imagine to create a master thesis on this question. But for that I start now with researching existing articles and papers on the topic of trust in the context of product design, UX and HCI. While researching keywords for the topic, I came across some scientific papers and articles available online, from which I want to sum up some interesting ideas here. These are only the first ideas I found, at the end I list up all publications that I found to be relevant to the topic as well.
Attributes of a product to build trust
In an article on uxdesign.cc about designing better products by building trust, Aimen Awan [1] mentions Erik Erikson’s stage model, where trust and mistrust is the first psychosocial development phase of a human being, until it has reached about 18 months of age. This period shapes their view of the world and their personality, so it is regarded as the most important period in a child’s life. [2] While the psychologists like Erikson see trust as a personal attribute and behavioral intention, there are other disciplines which handle the topic differently. Sousa, Dias and Lamas [4] describe the approach of computer scientists as observing trust as a rational choice against measurable risks. Further their second aspect of trust is the user’s cognition and affection, meaning the confidence in the system and their willingness to act. [4]
Awan further discusses the results of a study and experiment by P. Kulms and S. Kopp that people’s willingness to trust computer systems depend on the fundamental attributions of warmth and competence. When lacking the time and cognitive resources, people’s interpersonal judgements are mostly based on these two dimensions of social perception. [3]
Warmth can be described in HCI as confidence in the product, that it will help us reach a given goal. The overall user experience, design quality and visual consistency are largely influencing our perception of “warmth”, like transparent information display throughout the user’s journey with the product. E.g. if all details of a transaction are shown before decision making, we perceive the system as trustworthy and having good intentions. [1][3]
Competence is related to perceived intelligence – that a product can perform a given task accurately and efficiently. [1][3] As Awan mentions, Don Norman’s and Jacob Nielsen’s basic principles about usability represent the features of a product to be perceived as competent. Here Nielsen’s heuristics of “User freedom and control” are highlighted in particular. Unlike in human-human relationships, in HCI competence is not overruled by honesty, but is a crucial factor to build trust. [3]
She further discusses the importance of competence at the early stages of trust, depicted by expanding the trust pyramid model of Katie Sherwin. [5] In this new expanded concept, the foundational levels are the baseline relevance and trust that needs can be met and the interest and preference over other available options. These are definitely relying on the competence of the system and if these basic requirements are met, deeper trust can be built with personal and sensitive information (Level 3). From this level on the trust is deepened by perceived warmth, that could further lead to the willingness to commit to an ongoing relationship and even to recommendations to friends. [1] These stages may be more simple in the specific regard of automotive assistance systems, as in a car there aren’t several available options for the same task to choose from and only few tasks would require personal information. Nevertheless the concept can be relevant to an overall analysis of the topic.
Deriving design elements from theory to support trust
Four professors of the University of Kassel, Germany have made an experiment in 2012 on how to define Trust Supporting Design Elements (”TSDE”) for automated systems using trust theory. [6] They validated their findings through a laboratory experiment / user testing with 166 participants on a “context sensitive, self-adaptive restaurant recommendation system”, the “Dinner Now” app. Although this app has no similarities to driver assistance systems, the concept of deriving TSDEs could work generally.
Their motivation to write a work-in-progress paper was the often perceived lack of consideration of behavioral research insights in automation system design. There is potential to raise the achievable utility of products when behavioral truths are implemented into the development process. [6]
Here, the definition of trust by Lee and See [7] was highlighted as “the belief that an agent will help achieve an individual’s goal in a situation characterized by uncertainty and vulnerability”.
By applying the behavioral study concept of three identifiable dimensions of a user’s trust in automated systems (performance, process and purpose), Söllner et Al. created the following model of formation of trust (see Figure 1). The three dimensions are further based on indicators / antecedents [8], that cover different areas of the artifact and its relation to the user.
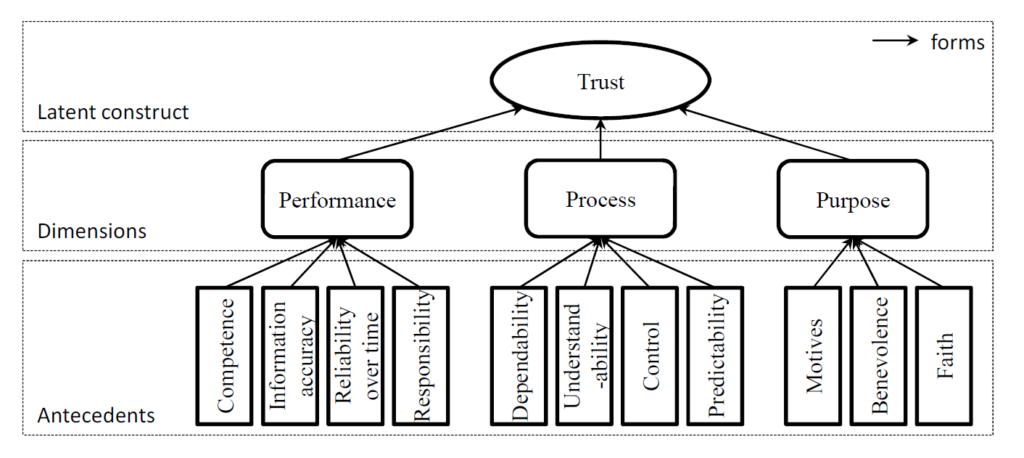
These antecedents are in short detail [8]:
- Competence – helping to achieve the user’s goal
- Information accuracy – of the presented information by the artifact
- Reliability over time
- Responsibility – the artifact having all functionalities to achieve the user’s goal
- Dependability – consistency of the artifacts behavior
- Understandability – how the artifact works
- Control – how much the user feels to have the artifact under control
- Predictability – anticipation of future actions of the artifact
- Motives – how well the purpose of the artifact’s designers is communicated to the user
- Benevolence – degree of positive orientation of the artifact towards the user
- Faith – general judgement, how reliable the artifact is
The paper describes a four-step model to systematically derive TSDEs from behavioral research insights (Figure 2) [6]:
- Identifying the uncertainties of the system that the user faces and
Prioritizing the uncertainties based on their impact - Choosing suitable antecedents to counter each uncertainty
- Interpreting and translating the antecedents into functional requirements
- Including these requirements into the design process and creating TSDEs
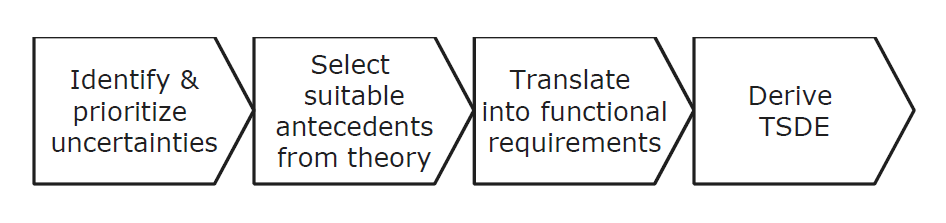
- In the case study, the specific uncertainties based on test-user prioritization were the quality of restaurant recommendations, the loss of control in the app and the reliability of user ratings.
- Thus the selected antecedents were understandability, control and information accuracy. For keeping developments costs in acceptable range, only one factor was considered for each uncertainty.
- From these antecedents, new requirements and features of the app were derived – like additional information to for more transparency, additional filtering possibilities for more control and friend’s ratings option for more reliability.
The final user studies and questionnaires resulted in the validation of the model to be effective and suitable to derive valuable design elements – the TSDEs were appreciated by the participants and the trust and chances of future adoption of the app were enhanced. [6]
To enhance in-vehicle user interfaces a similar approach could be applied to find helpful solutions strengthening the trust in the system.
Building trust in self-driving technology
In 2020, Howard Abbey, an autonomous car specialist ar SDB Automotive gave a presentation on “How Can Consumers Understand the Difference Between Assisted and Autonomous Driving?”. Emily Pruitt summed up the five key takeaways of this talk, how to increase the user’s understanding and adoption of ADAS systems. [9]
- Design out potential misuse
Users will push the limits of reasonable safety of automated systems. Therefor the systems have to be designed in a way to prohibit any misuse possibility. E.g. warn the driver if hands are off the steering wheel or eyes are not on the road, or stop self-parking assistance when doors get opened. It has to be clarified for the user, what is assistance and what is autonomous. - Use common naming
Safety critical features should have naming conventions across different OEM platforms. As long as there are different descriptions for similar systems, the driver cannot rely on their previous experiences and has to learn the systems in case of change of vehicles again and again. (Currently there are 100+ names for emergency braking, 77 for lane departure, 66 for adaptive cruise control and 57 for blind spot monitoring. Though progress is already made by SAE International together with other organisations to recommend common naming, so that drivers can be educated on the same fundamentals) - Be clear
SDB Automotive carried out a user study on driver interaction with HMI systems – assigning them tasks to use assistants and and measuring completion time and mental workload. The assessment was done in regard to differences in HMI systems of several manufacturers. Results show three issues that lead to comprehension difficulties when finding the right system, engaging it and reading its feedback:- confusing display graphics
- unclear system status
- inconsistent icons
- Unify Systems
Several industry experts believe that ADAS systems should be simplified or combined if possible, as the number of seemingly similar systems is growing. Drivers shouldn’t think about the functionality of systems to choose for the specific situation, instead of focusing on the road. One holistic overall system should work in the background and “take care of the complexity for the user”. - Give simple choice
Within the holistic system, there is no need to let the driver choose from seemingly similar systems and get confused (e.g. cruise control vs. automated cruise control vs. traffic jam assist). The options should be held simple with driving states: manual, mixed or autonomous.
[9]
Further questions
Further questions arise if we think about state-of-the-art (2022) and future technologies – also with regard to the possibilities of multimodal interaction and augmented reality.
- Are the further above mentioned antecedents applicable for fully automated, safety critical systems and are there further ones?
- How can we find the most suitable design solutions to fulfill the specific requirements to build more trust?
- What augmentation technologies apply the best as additional solutions? Visual, sound or haptic feedbacks, or all of them?
- Vehicles can be used for many tasks. Are there different use cases with special uncertainties to be consider?
- Vehicles’ user groups vary a lot. Are there design solutions that can fulfill requirements for different use cases and user groups?
- What different trust aspects arise when the automated system is equipped with Artificial Intelligence?
During my research per date I found many more scientific publications that are of interest and have to be read next. I hope to find material to be able to answer these questions. I just found a master thesis from the Chalmers University of Technology written in 2020 (see at the bottom of the list below) that already discusses my proposed topic very similarly. So further on I have to focus on the still to be researched areas to build my master thesis on, like probably the AR implementations in regard of the trust issues.
Literature sources to consider further:
- Söllner, M.; Hoffmann, A.; Hoffmann, H. & Leimeister, J. M. (2011): Towards a Theory of Explanation and Prediction for the Formation of Trust in IT Artifacts. In: 10. Annual Workshop on HCI Research in MIS, Shanghai, China.
- Lee, J. D. and See, K. A. (2004) ‘Trust in Automation: Designing for Appropriate Reliance’, Human Factors, 46(1), pp. 50–80. doi: 10.1518/hfes.46.1.50_30392.
- Hoff, K. A. and Bashir, M. (2015) ‘Trust in Automation: Integrating Empirical Evidence on Factors That Influence Trust’, Human Factors, 57(3), pp. 407–434. doi: 10.1177/0018720814547570.
- Parasuraman, R. and Riley, V. (1997) ‘Humans and Automation: Use, Misuse, Disuse, Abuse’, Human Factors, 39(2), pp. 230–253. doi: 10.1518/001872097778543886.
- Molina, M. D. and Sundar, S. S. (2022) ‘Does distrust in humans predict greater trust in AI? Role of individual differences in user responses to content moderation’, New Media & Society. doi: 10.1177/14614448221103534.
- Dixon L., Megill W., Nebe K. (2019).: Trust in Automation: An On-Road Study of Trust in Advanced Driver Assistance Systems. In: VEHICULAR 2019: The Eighth International Conference on Advances in Vehicular Systems, Technologies and Applications, Rome, Italy
- DeGuzman C. A., Donmez B. (2021): Knowledge of and trust in advanced driver assistance systems, Accident Analysis & Prevention, Volume 156, 106121, ISSN 0001-4575, https://doi.org/10.1016/j.aap.2021.106121. (https://www.sciencedirect.com/science/article/pii/S0001457521001524)
- Steven D. Lubkowski, Bridget A. Lewis, Valerie J. Gawron, Travis L. Gaydos, Keith C. Campbell, Shelley A. Kirkpatrick, Ian J. Reagan, Jessica B. Cicchino: Driver trust in and training for advanced driver assistance systems in Real-World driving, Transportation Research Part F: Traffic Psychology and Behaviour, Volume 81, 2021, Pages 540-556, ISSN 1369-8478, https://doi.org/10.1016/j.trf.2021.07.003. (https://www.sciencedirect.com/science/article/pii/S1369847821001625)
- Fjollë Novakazi, Julia Orlovska, Lars-Ola Bligård, Casper Wickman: Stepping over the threshold linking understanding and usage of Automated Driver Assistance Systems (ADAS), Transportation Research Interdisciplinary Perspectives, Volume 8, 2020, 100252, ISSN 2590-1982, https://doi.org/10.1016/j.trip.2020.100252. (https://www.sciencedirect.com/science/article/pii/S2590198220301639)
- Bo Yu, Shan Bao, Yu Zhang, John Sullivan, Mike Flannagan: Measurement and prediction of driver trust in automated vehicle technologies: An application of hand position transition probability matrix, Transportation Research Part C: Emerging Technologies, Volume 124, 2021, 102957, ISSN 0968-090X, https://doi.org/10.1016/j.trc.2020.102957. (https://www.sciencedirect.com/science/article/pii/S0968090X20308548)
- Maryam Zahabi, Ashiq Mohammed Abdul Razak, Ranjana K. Mehta, Michael Manser: Effect of advanced driver-assistance system trainings on driver workload, knowledge, and trust, Transportation Research Part F: Traffic Psychology and Behaviour, Volume 76, 2021, Pages 309-320, ISSN 1369-8478, https://doi.org/10.1016/j.trf.2020.12.003. (https://www.sciencedirect.com/science/article/pii/S136984782030588X)
- Julia Orlovska, Fjollë Novakazi, Bligård Lars-Ola, MariAnne Karlsson, Casper Wickman, Rikard Söderberg: Effects of the driving context on the usage of Automated Driver Assistance Systems (ADAS) -Naturalistic Driving Study for ADAS evaluation, Transportation Research Interdisciplinary Perspectives, Volume 4, 2020, 100093, ISSN 2590-1982, https://doi.org/10.1016/j.trip.2020.100093. (https://www.sciencedirect.com/science/article/pii/S259019822030004X)
- Weast J., Yurdana M., Jordan A. (2016): A Matter of Trust: How Smart Design Can Accelerate Automated Vehicle Adoption. Intel Corporation. https://www.intel.com/content/dam/www/public/us/en/documents/white-papers/trust-autonomous-white-paper-secure.pdf
- Anthony C (2020): PAVE STUDY & RESEARCH LENDS INSIGHT INTO HOW CONSUMERS VIEW AUTONOMOUS VEHICLES Article on AutoVisionNews. https://www.autovision-news.com/industry/pave-study/
- Shi, Yuan & Azzolin, Nicolò & Picardi, Andrea & Zhu, Tao & Bordegoni, Monica & Caruso, Giandomenico. (2020). Validating HMI Design for Increasing User’s Trust in Autonomous Vehicle. 237-241. 10.14733/cadconfP.2020.237-241.
- Master Thesis: Fredrick Ekman, Mikael Johansson, Jana L. Sochor (2016): CREATING TRUST IN AUTOMATED VEHICLES USING A HMI-BASED FRAMEWORK; Published in: The 5th Swedish National Conference on Transport Research, Lund, Sweden, October 18-19, 2016 http://publications.lib.chalmers.se/publication/244498-creating-trust-in-automated-vehicles-using-a-hmi-based-framework https://publications.lib.chalmers.se/records/fulltext/226342/226342.pdf
Sources
[1] Awan A. (2019): Design better products by building trust; article on uxdesign.cc, retrieved on 10.07.2022 from: https://uxdesign.cc/design-better-products-by-building-trust-94639617c81
[2] Cherry K. (2021): Trust vs. Mistrust: Psychosocial Stage 1; article on verywellmind.com; retrieved on 11.07.2022 from: https://www.verywellmind.com/trust-versus-mistrust-2795741
[3] Kulms P., Kopp S. (2018): A Social Cognition Perspective on Human–Computer Trust: The Effect of Perceived Warmth and Competence on Trust in Decision-Making With Computers. Front. Digit. Humanit. 5:14. doi: 10.3389/fdigh.2018.00014 retrieved on 11.07.2022 from: https://www.frontiersin.org/articles/10.3389/fdigh.2018.00014/full
[4] Sousa S. C., Dias P., Lamas D. (2014) A Model for Human-Computer Trust; retrieved on 08.07.2022 from: https://www.researchgate.net/publication/266087967_A_Model_for_Human-Computer_Trust
[5] Sherwin K. (2016): Hierarchy of Trust: The 5 Experiential Levels of Commitment; Nielsen Norman Group; retrieved on 13.07.2022 from: https://www.nngroup.com/articles/commitment-levels/
[6] Söllner, M.; Hoffmann, A.; Hoffmann, H. & Leimeister, J. M. (2012): How to Use Behavioral Research Insights on Trust for HCI System Design. In: ACM SIGCHI Conference on Human Factors in Computing Systems (CHI), Austin, Texas, USA retrieved on 09.07.2022 from: https://www.researchgate.net/publication/254005515_How_to_use_behavioral_research_insights_on_trust_for_HCI_system_design
[7] Lee, J.D. and See K.A. (2014): Trust in Automation: Designing for Appropriate Reliance. Human Factors 46, 1, 50-80. retrieved on 14.07.2022 from: https://journals.sagepub.com/doi/abs/10.1518/hfes.46.1.50_30392
[8] Söllner, M.; Hoffmann, A.; Hoffmann, H. & Leimeister, J. M. (2011): Towards a Theory of Explanation and Prediction for the Formation of Trust in IT Artifacts. In: 10. Annual Workshop on HCI Research in MIS, Shanghai, China.
[9] Pruitt E. (2020): HOW CAN OEMS BUILD CONSUMER TRUST IN SELF-DRIVING TECHNOLOGY?, Article on AutoVisionNews. retrieved on 14.07.2022 from: https://www.autovision-news.com/adas/consumer-trust-self-driving-technology/